Contact:
- email:
- bartosz.czaplewski@pg.edu.pl
Positions:
Assistant professor
- workplace:
- Katedra Sieci Teleinformacyjnych
Budynek A Wydziału Elektroniki, Telekomunikacji i Informatyki, EA 604
- phone:
- (58) 347 11 61
Functions:
Deputy Head of Department
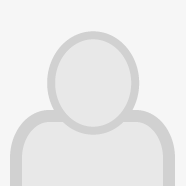
Publications:
-
Publication
- Year 2024
Forward error correction is crucial for communication, enabling error rate or required SNR reduction. Longer codes improve correction ratio. Iterated codes offer a solution for constructing long codeswith a simple coder and decoder. However, a basic iterative code decoder cannot fully exploit the code’s potential, as some error patterns within its correction capacity remain uncorrected.We propose two neural network-assisted decoders:...
Full text to download in external service
-
Publication
- A. Bekasiewicz
- V. Waladi
- T. Dhaene
- B. Czaplewski
- Year 2024
Antenna far-field performance is normally evaluated in expensive laboratories that maintain strict control over the propagation environment. Alternatively, the responses can be measured in non-anechoic conditions and then refined to extract the information on the structure field-related behavior. Here, a framework for correction of antenna measurements performed in non-anechoic test site has been proposed. The method involves automatic...
Full text to download in external service
-
Publication
- Year 2024
This paper investigates the impact of various modifications introduced to current state-of-the-art Convolutional Neural Network (CNN) architectures specifically designed for the steganalysis of digital images. Usage of deep learning methods has consistently demonstrated improved results in this field over the past few years, primarily due to the development of newer architectures with higher classification accuracy compared to...
Full text to download in external service
-
Publication
- ISA TRANSACTIONS - Year 2022
The article concerns the automation of vessel movement anomaly detection for maritime and coastal traffic safety services. Deep Learning techniques, specifically Convolutional Neural Networks (CNNs), were used to solve this problem. Three variants of the datasets, containing samples of vessel traffic routes in relation to the prohibited area in the form of a grayscale image, were generated. 1458 convolutional neural networks with...
Full text available to download
-
Publication
Nematodes Caenorhabditis elegans (C. elegans) have been used as model organisms in a wide variety of biological studies, especially those intended to obtain a better understanding of aging and age-associated diseases. This paper focuses on automating the analysis of C. elegans imagery to classify the muscle age of nematodes based on the known and well established IICBU dataset. Unlike many modern classification methods, the proposed...
Full text available to download
Projects:
-
Projects
Project manager: dr hab. inż. Sylwester Kaczmarek Financial Program Name: OBRONNOŚĆ I BEZPIECZEŃSTWO
Project realized in Department of Teleinformation Networks according to DOB-BIO6/10/62/2014 agreement from 2014-12-23